Secure Machine Learning Architecture
With SEMLA we develop a demand driven infrastructure to be able to work with extremely sensitive personal data in research projects.
Read more in our white paper.
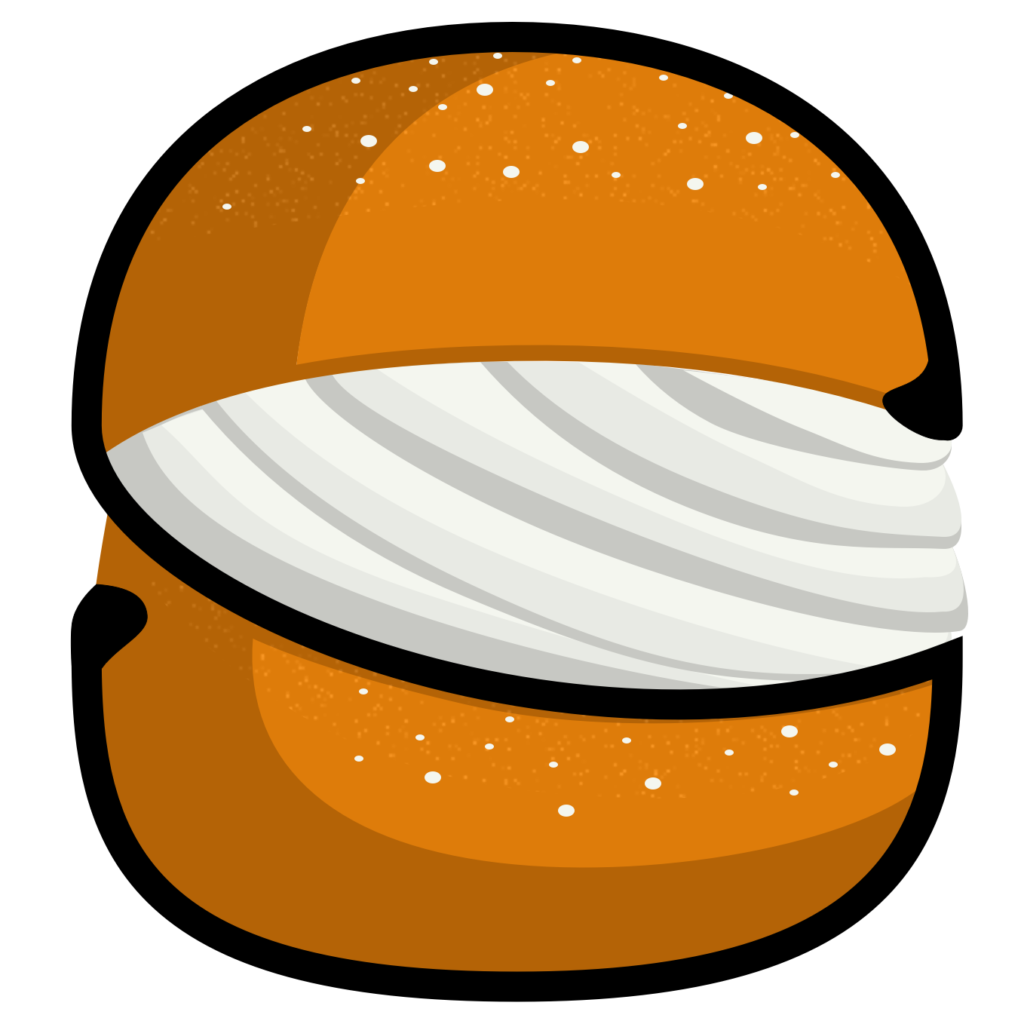
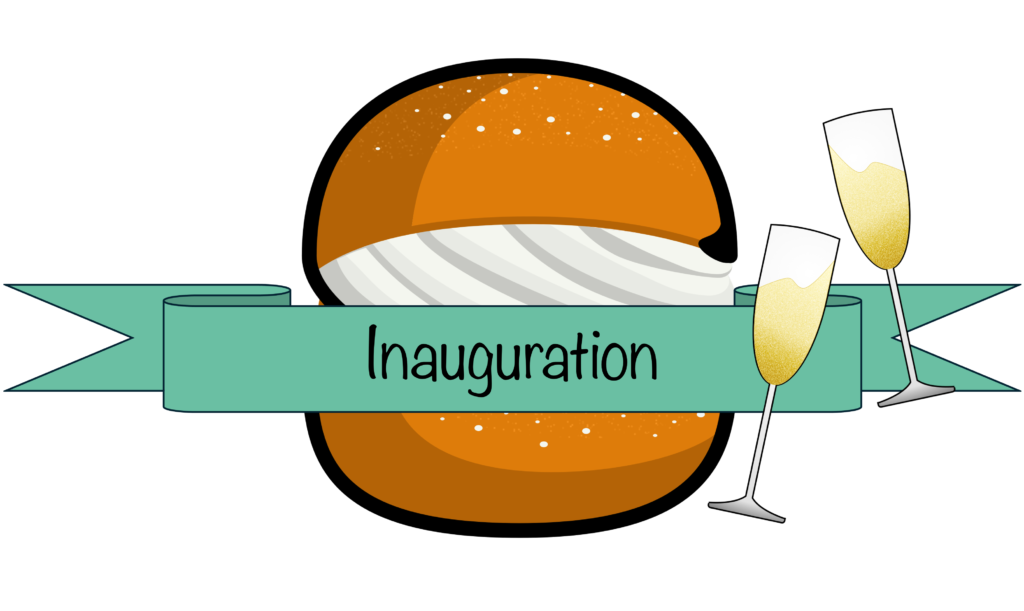
SEMLA is inaugurated
SEMLA and especially SEMLab were officially opened and pronounced to the whole DFKI and the world.
Data Classification
SEMLA uses six sensitivity classes to classify the data and apply corresponding TOMs — technical and organizational measures — measures, ranging from open accessible public data without any restrictions to extra sensitive personal data that can only be accessed from strongly restricted offices.
The highest sensitivity level is reserved for extremely sensitive data whose exceptional security requirements cannot be met by SEMLA.
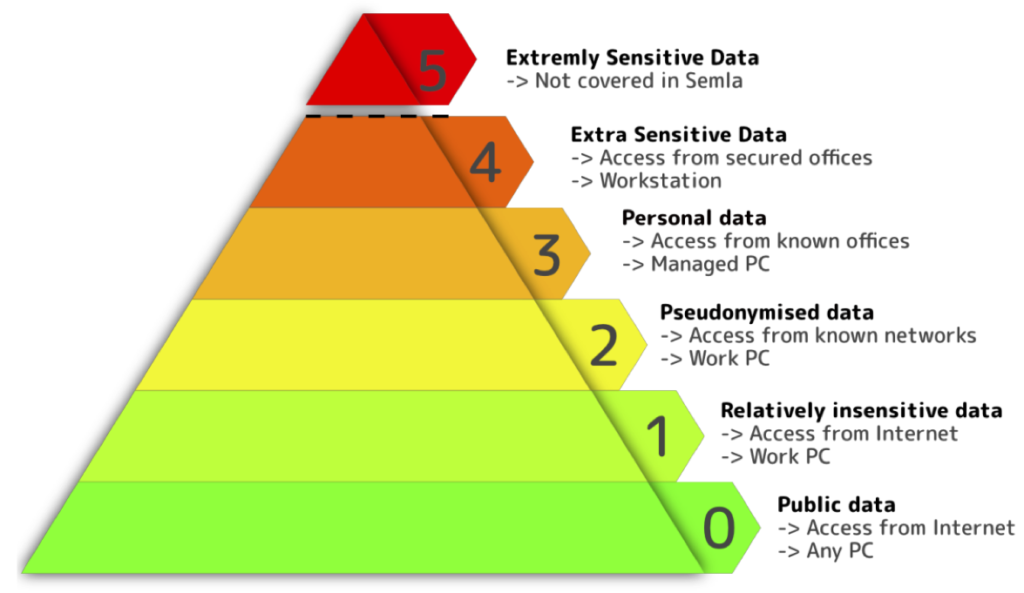
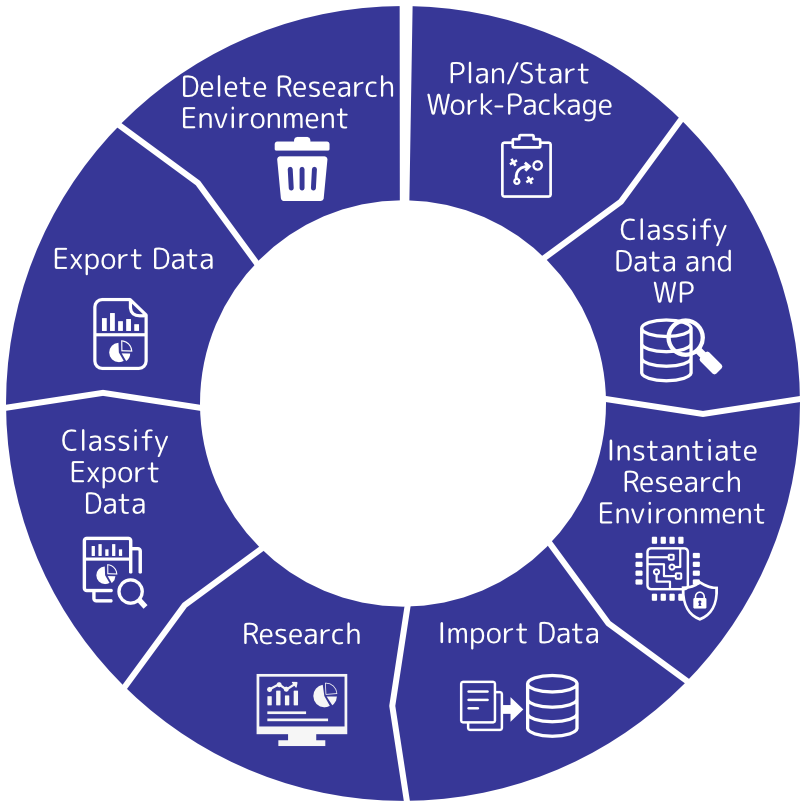
Data Life Cycle
SEMLA follows a strict data life cycle that handles all the steps from planning data-based research, over data classification and import until data export and deletion.
Encapsulation
To ensure compartmentalization and multi-tenancy, every Work package — a group of data sets with a fixed intended use, result data and a corresponding work environment — is encapsulated in multiple ways, ranging from single purpose VMs, over encrypted storage containers and secured communication to separated networks.
These fine-grained encapsulation methods pave the way for conditional access restrictions per work package according to their sensitivity level.
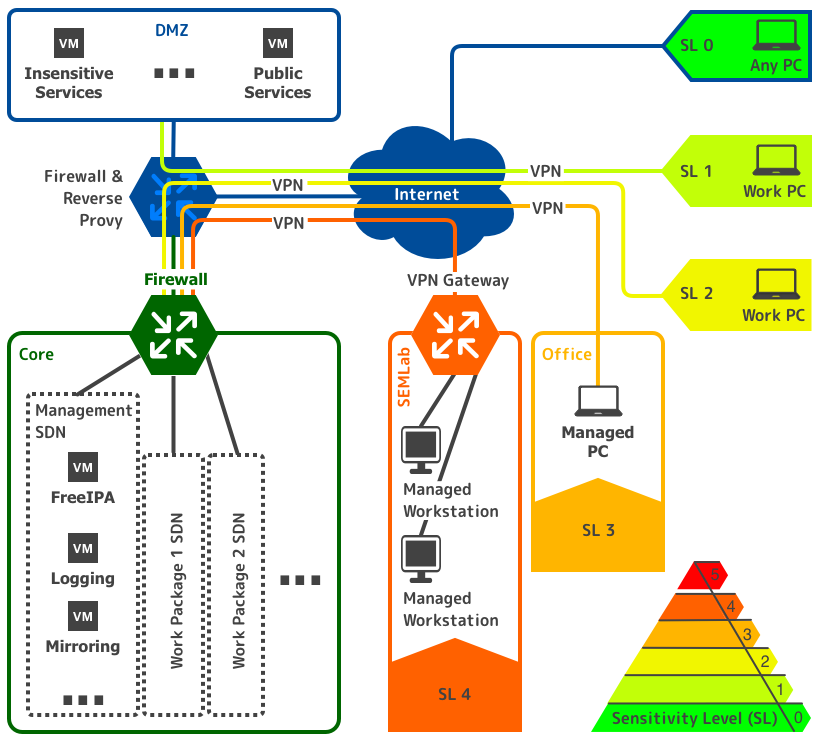
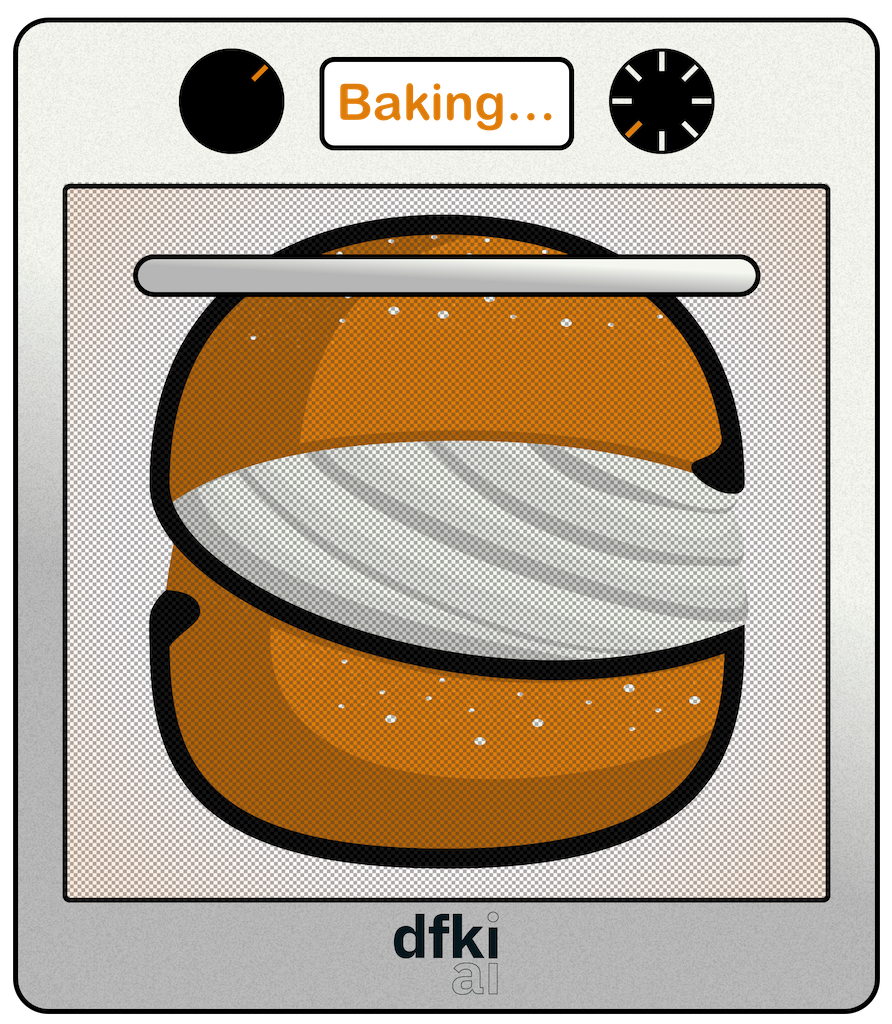
SEMLA is baking …
SEMLA and it’s website is under extensive development and since it is demand driven, it will constantly evolve.
More and more content, tools, documentation and source code will be made available over time.